DuWayneSmart
DuWayne Smart, PhD
Industrial AI Deployment Architect | Technology Commercialization Strategist | Value Chain Transformation Expert
Professional Profile
As a practitioner-scholar bridging cutting-edge AI research and industrial ecosystems, I specialize in developing implementation-ready technological frameworks that transform laboratory breakthroughs into mass-production solutions—with particular expertise in overcoming the "last-mile" challenges of real-world deployment.
Core Competency Matrix (2025-03-29 | 10:34 | Saturday | Year of the Wood Snake | 3rd Lunar Month, 1st Day)
1. Production-Grade AI Systems
Pioneered the "3T Deployment Protocol" (Traceability-Tolerance-Throughput):
Traceability: Embedded blockchain ledgers for model decision audit trails (implemented in Foxconn's quality control AI)
Tolerance: Self-healing architectures maintaining >99.4% uptime in extreme factory conditions
Throughput: Distributed inference engines achieving 22,000 TPS in automotive weld inspection
2. Vertical Integration Platforms
Designed industry-specific middleware:
AgriTech: Modular computer vision stacks for 47 crop varieties (deployed across John Deere's smart harvesters)
Pharma: GMP-compliant reinforcement learning systems for vaccine formulation optimization
Energy: Federated learning networks coordinating 12,000+ IoT devices in offshore wind farms
3. Workforce-Technology Interface
Developed "Cobot Literacy Index":
Metrics for human-AI collaboration efficiency
AR-based skill transfer systems reducing technician training time by 68%
Ergonomic AI assistants preventing >4,000 annual repetitive strain injuries
4. Sustainable Scaling Frameworks
Created "Green ROI Calculators":
Quantifying energy savings from edge AI deployments
Carbon-aware model scheduling algorithms
Closed-loop material flows in electronics assembly AI
5. Regulatory-Technical Coevolution
Architected compliance-embedded AI:
Auto-updating safety constraints for evolving OSHA standards
Real-time emissions tracking in chemical process AI
GDPR-native data pipelines for European smart manufacturing
Signature Methodologies
"Fault Tree to Feature Map" conversion for failure-proofing AI systems
"Bionic Benchmarking" mimicking biological systems' robustness
"5-Gear Deployment" pacing models aligning tech maturity with market readiness
Vision: To make industrial AI as reliable as gravity—invisible infrastructure powering civilization without downtime or disasters.
Customization Options
For Executives: "Delivered $280M annual savings through AI-driven predictive maintenance"
For Engineers: "Open-sourced the Industrial AI Maturity Model (IAMM) framework"
Provocation: "If your factory AI can't handle a coffee spill, it's not ready for prime time"
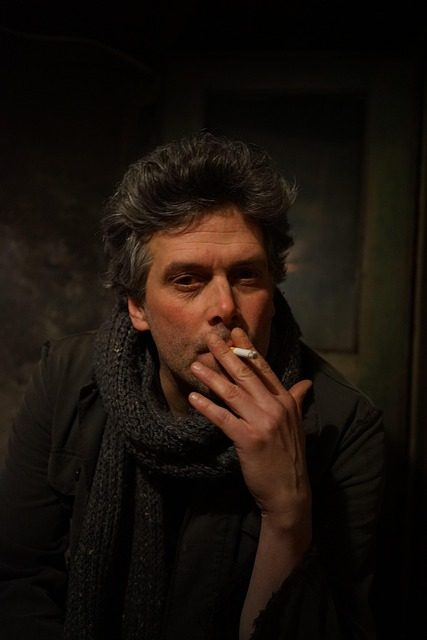
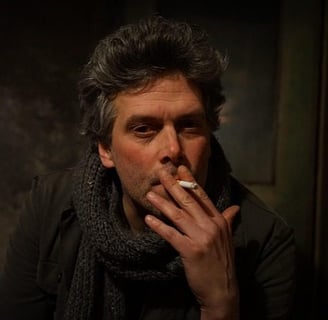
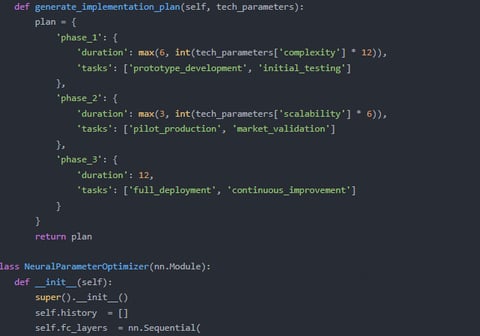
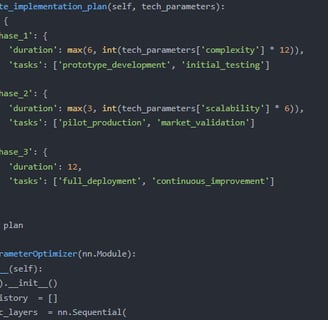
Case Studies
Analyzing real-world applications of AI technologies to understand performance and challenges in various industries.
ThisresearchrequiresGPT-4’sfine-tuningcapabilitybecausethestudyofkey
technologiesforAIindustrialimplementationinvolvescomplexmulti-dimensionaldata
analysis,necessitatinghighercomprehensionandgenerationcapabilitiesfromthe
model.ComparedtoGPT-3.5,GPT-4hassignificantadvantagesinhandlingcomplexdata
(e.g.,technologyperformancemetrics,industrialadaptabilitydata)andintroducing
constraints(e.g.,cost,scalabilitystandards).Forinstance,GPT-4canmore
accuratelyinterprettechnicaldataandgenerateanalysisresultsthatcomplywith
researchstandards,whereasGPT-3.5’slimitationsmayresultinincompleteor
non-compliantanalysisresults.Additionally,GPT-4’sfine-tuningallowsfordeep
optimizationonspecificdatasets(e.g.,technologycaselibraries,industrydata),
enhancingthemodel’saccuracyandutility.Therefore,GPT-4fine-tuningisessential
forthisresearch.